Avoiding Conflicts on Social Media Might Make Things Worse
Look, I get it. Sometimes you really don’t want to get mired in a Facebook discussion with that uncle of yours who thinks that the moon doesn’t exist. It’s easier to block, unfriend, ignore, rather than engage. However, have you considered that avoiding conflicts might make things worse? This is a question Luca Rossi and I asked ourselves as a part of our research on polarization on social media. Part of the answer comes from an Agent Based Model (ABM) we have recently published on Plos One in the paper “How Minimizing Conflicts Could Lead to Polarization on Social Media: an Agent-Based Model Investigation.”
Specifically, we were interested in looking at how news sources react when they get attacked on social media with backlash and flagging. This is a followup to our previous paper, where we found — surprisingly — that this backlash and flagging is mostly directed at neutral and factual news sources. The reason why these sources are magnets for controversies is because their stories are widely read, and thus attract the ire of all sorts of quacks. Quackery, instead, is only read by quacks agreeing with it, and thus they don’t quack at it so much.
So now the question is: what does this negative attention from quacks do to a neutral news source? To answer the question we updated our ABM. In the original version, each news source and user had a fixed political position — a numerical value between -1 (extreme left) and +1 (extreme right), with 0 as perfect neutrality. In the new version, their position can change. People get attracted by similar points of view and repulsed by opinions that are too far from their position. For instance, a +1 user might get attracted if they read a +0.9 news item (moving to, say, a +0.95 position), but will be repulsed again if they read a -0.5 item next (moving back to, say, a +0.98 position).
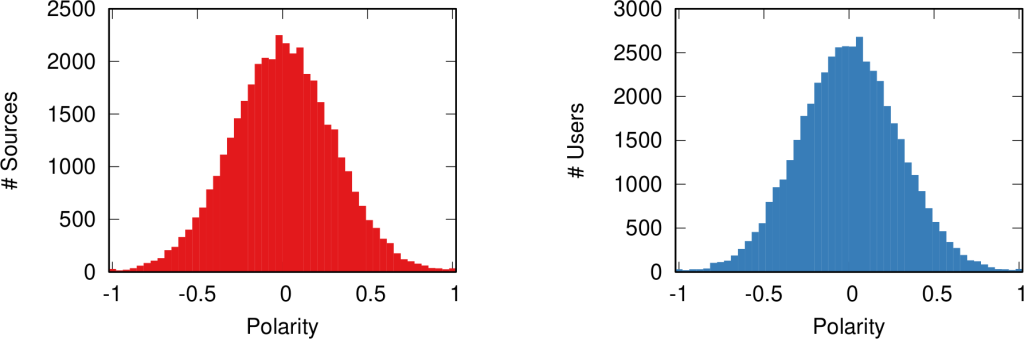
If a user is repulsed, they will also flag the news source. A news source doesn’t want to be flagged. A flag is a bad omen: too many flags and the news source might get banned by the social media platform, or be subject to big scary fact-checking banners. They might even — gasp! — make Mark Zuckerberg leak a tear. Social media are too important for news sources to let this happen. So they will try to avoid conflict. Since they know flags come from people with a different opinion from their own, the only thing they can do is to change their stance. The safest bet is to average the opinions of all their readers. Taking the average of their neighbors in most cases would lead to settle in the middle of the polarity spectrum, but this is not guaranteed.
Four factors together create the rules of the game: how much users feel the need to share new articles on social media; how tolerant they are with diverse viewpoints; how much news sources will try to resist the pressure to change their spin; and how quickly users change their own opinion following what they read. Having an ABM allows you to run a lot of simulations and see what the effect of each of this aspect is on the final system.
We find that:
- The more people share, the more news sources will be pushed away from neutrality and become partisan;
- The less tolerant users are, the more they will increase polarization;
- The resistance a news organization puts up against such a pressure is irrelevant to the final state of the system;
- If users change their opinions easily, they will be attracted to the extreme ends of the polarity spectrum.
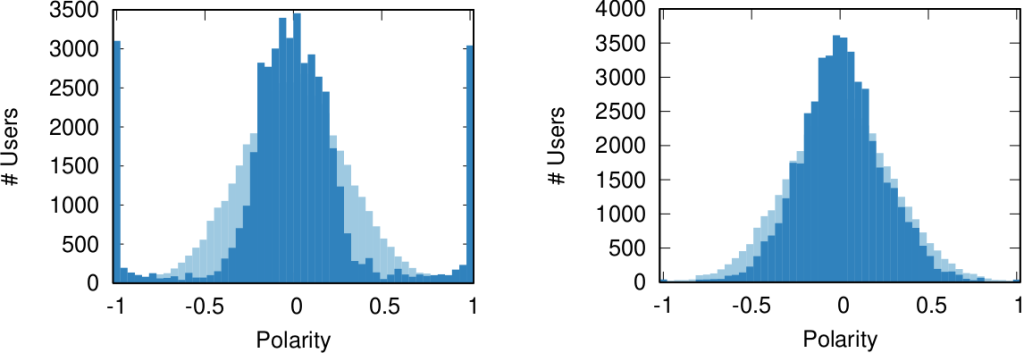
Some of this is unsurprising — intolerance breeds polarization –, while other things might be worth looking at a second time. For instance, we think polarization is a bigger deal nowadays exactly because social media helps sharing in a way newspapers, radio, and television do not. Our results say that this oversharing exacerbates polarization. But a nagging question remains: is our ABM just a theoretical toy, or can it reproduce reality?
We think the latter is true, because we tested it against a real world Twitter network. We have a network topology of who talks with whom, and a polarity score for each user based on the news sources they cite in their tweets. The parameter combination that best reproduces real world data is this: high sharing, high opinion volatility, and low tolerance. Which in our model is the exact recipe for escalating conflict. And all of this just because news sources eschew conflict and don’t want to be flagged. Ouch.
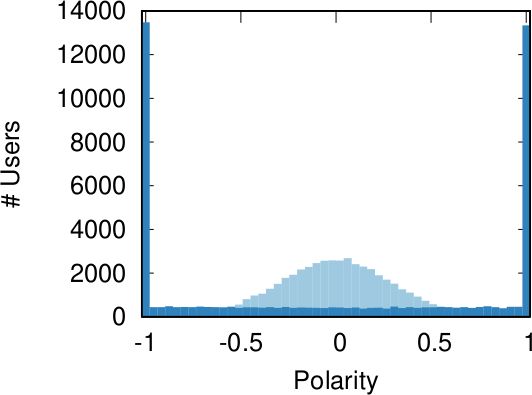
The same grains of salt that you should take our previous paper with also apply here. The model is based on assumptions, and thus it is only as good as those assumptions. Moreover, reality is more complicated than our ABM. For instance, we assume tolerance is uncorrelated with one own political opinion. But what if some political opinions tend to go together with being less tolerant of other points of view? And what if users don’t genuinely flag what they think is outrageous, but make a more strategic use of the flag button to advance their own agenda? These are questions we will explore in further developments of our work.